UNN-GazeEAR: An Activities of Daily Life Dataset for Egocentric Action Recognition
2 Faculty of Computer Science, University of Sunderland, Sunderland, U.K.
3 Cognitive Science Department, Xiamen University, Xiamen, China
4 Information Science and Technology College, Dalian Maritime University, Dalian, China
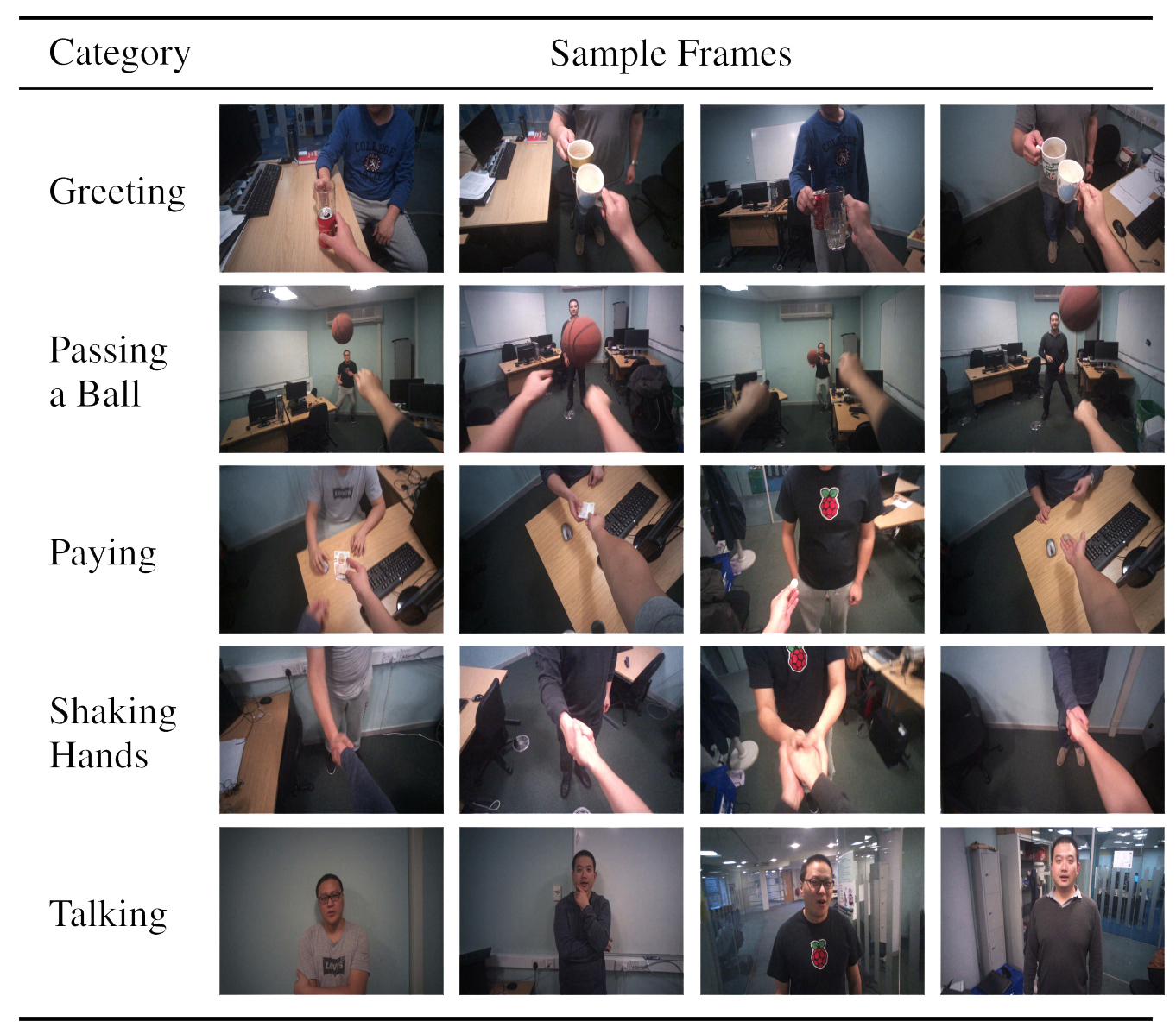
Introduction
The UNN-GazeEAR includes original set and gaze-region-of-interest (GROI) set, with some sample frame are visualised above. Each set contains 50 human-to-human interaction video clips (10 clips per class) in 7,586 frames in total that collected using Tobii Pro® Glasses 2. Each video clip is of the same resolution of 1920-by-1080 pixels with a unified frame rate of 25 frames per second (FPS) but with different time duration ranging from 2 to 11 seconds. To ease the computational cost, all the video clips in the dataset have been resized to 320-by-180 pixels. The data set is associated with frame-wise gaze point which is determined based on a built-in eye fixation analysis function, which in turn results in the frame-wise GROI using our algorithm (visualised on the right side of the figure below).
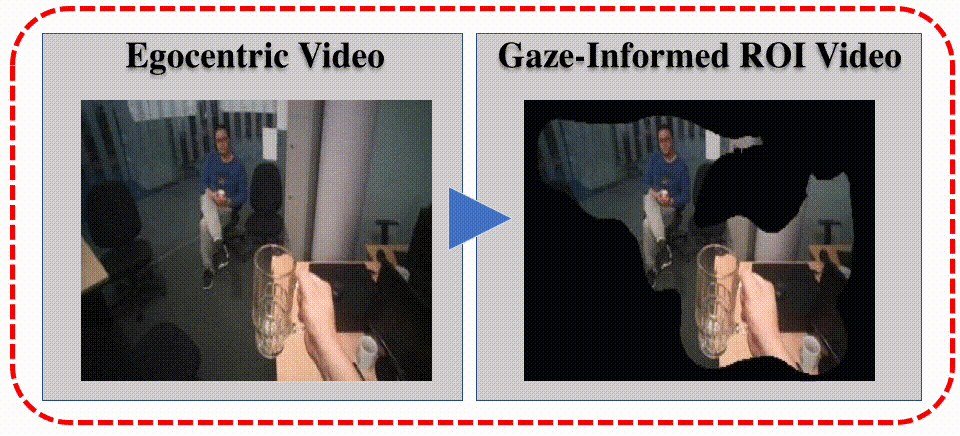
Download
- UNN-GazeEAR Dataset
- contains both UNN-GazeEAR Original Set and UNN-GazeEAR GROI Set (73 MB)
- [Google Drive]
- UNN-GazeEAR Original Set
- contains the original set merely (45 MB)
- [Google Drive]
- UNN-GazeEAR GROI Set
- contains the GROI set merely (27 MB)
- [Google Drive]
- The Five ADL Set
- contains the original and its GROI version of an untrimmed video clip that consists of 5 consecutive human actitiveies (3 MB)
- optional in the testing phase of the experiments
- [Google Drive]
Acknowledgement
This work was supported by the National Natural Science Foundation of China under Grant 61502068. We also like to thank Jie Li and Noe Elisa Nnko for their participations in data collection.
Citation
If you use this datset in your research, please refer to the following paper:
@ARTICLE{zuo18EgoActionRecognit, author={Z. {Zuo} and L. {Yang} and Y. {Peng} and F. {Chao} and Y. {Qu}}, journal={IEEE Access}, title={Gaze-Informed Egocentric Action Recognition for Memory Aid Systems}, year={2018}, volume={6}, number={}, pages={12894-12904}, doi={10.1109/ACCESS.2018.2808486}, ISSN={2169-3536}, month={},}
For more experimental results on the UNN-GazeEAR dataset, please refer to the following works:
@INPROCEEDINGS{zuo18SstVladSstFv, author = {Zheming Zuo and Daniel Organisciak and Hubert P. H. Shum and Longzhi Yang}, title = {Saliency-Informed Spatio-Temporal Vector of Locally Aggregated Descriptors and Fisher Vectors for Visual Action Recognition}, booktitle = {2018 BMVA British Machine Vision Conference (BMVC)}, pages = {321.1--321.11}, year = {2018}}
@ARTICLE{zuo2019EnhancedLFD, title={Enhanced gradient-based local feature descriptors by saliency map for egocentric action recognition}, author={Zuo, Zheming and Wei, Bo and Chao, Fei and Qu, Yanpeng and Peng, Yonghong and Yang, Longzhi}, journal={Applied System Innovation}, volume={2}, number={1}, pages={7}, year={2019}, publisher={Multidisciplinary Digital Publishing Institute},}